CHALLENGE: Economically scale-up specialty plastics formulations from R&D scale to production scale
SOLUTION: Develop customized active learning-based optimization tool to recommend measurement conditions to scale-up operators
RESULTS: The optimization tool is projected to save $90,000 per formulation scale-up, significantly reduce the risk of scale-up failure, and shorten the time to market for new specialty plastics by several months.
A Specialty Chemical Company Wanted to Remove the Bottleneck from their Polymer Scale-Up Process
Many of the high tech products we interact with on a daily basis are enabled by the use of specialty plastics that are carefully engineered to have a wide range of critical properties (mechanical, optical, electrical, thermal) that must be co-optimized to meet target performance requirements, all while taking a variety of costs into consideration. These high-performance products are created using a complex formulation of custom polymer chemistries, chemical additives, fillers, colorants, and more. Product creation is further complicated by the fact that the final properties can strongly depend on how these formulations are melted and mixed together. However, even once desirable recipes and process conditions are identified in the laboratory, the work of producing this material for end-use products is not complete.
The next hurdle in the process is to scale up the formulation to production-level quantities. Scale-up is the phase of R&D where a material produced at a small scale is reproduced at a much larger scale for production. Economically producing these materials at-scale requires the use of much larger mixing and extrusion systems than those found in laboratories. These production mixers can significantly alter the temperature and physical forces imparted to the material during processing. However, production-scale tests are very expensive in terms of time and hard costs.
One common way to match properties between the lab-scale and the production scale is to try to match the amount of mechanical energy applied to the material during processing. While there are a number of traditional experimental design strategies that can help make this matching process reasonably efficient and successful, scale-up efforts often fail to reach target performance on the first try and require multiple attempts to be in the performance window. With tight experimental budgets, the process is a major bottleneck in developing and commercializing new materials.
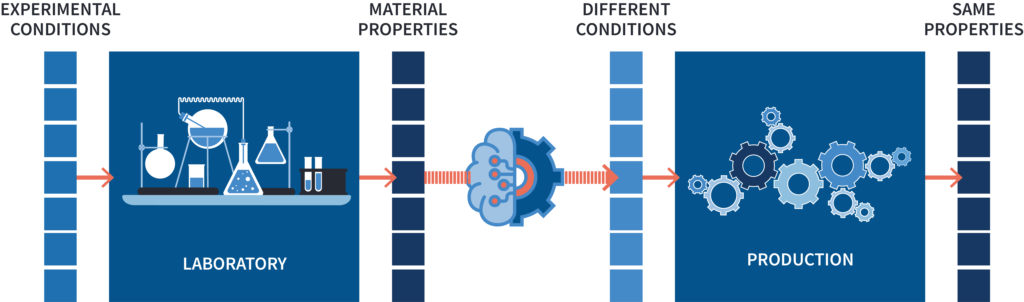
Scale-up can be framed as the problem of finding input parameters under varying conditions that produce the same output results.
Lab Transformation, and a New Workflow Designed to Leverage Automation and Digitization.
To more efficiently make use of limited experimental budgets, we worked closely with client R&D scientists to revamp their scale-up workflow and integrate their domain expertise into a customized active learning-based Bayesian optimization tool. In this workflow, experimental data at the laboratory scale, historical scale-up data, and expert domain knowledge are used to determine an initial experimental design for production tests and construct a rough initial estimate for the mechanical energy response function. Once production tests begin, the active learning tool then charts a course through possible processing conditions to quickly find the desired mechanical energy mixing condition. As each trial is completed, the tool fits a Gaussian process regression model to the data, and iteratively learns the true relationships. Based on where the model predicts the target is located and the uncertainties associated with these predictions, the tool adjusts the course as needed. In this way, the tool minimizes the number of exploratory steps required to find working scale-up parameters.
The resulting solution integrates deep domain expertise and modern machine learning techniques into a simple, deployable application that can be easily used by scale-up operators on the production floor.
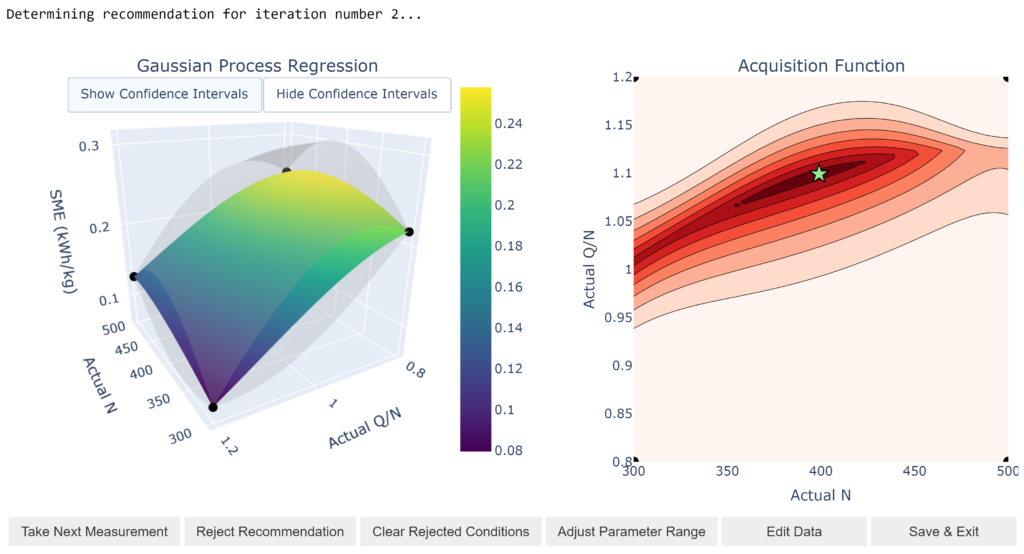
The images above are screenshots of the interactive data visualizations in the active learning software tool developed for the client. (Left) Gaussian process regression was used to fit the data and determine areas of uncertainty. The colored surface represents the best fit and the translucent grey surfaces show the upper and lower confidence bounds. The app uses this technique to balance the desire to explore the parameter space with the need to efficiently find the target. (Right) A contour plot of the acquisition function determines where the algorithm recommends the next measurement. The “green star” marks the app’s recommendation for the next measurement for the scientist to investigate.
Risk of Scale-Up Failure and Time-to-Market Are Significantly Reduced
The scale-up optimization tool decreases the number of experiments needed to reach the target mechanical energy mixing condition by over 50% compared to traditional methods and produces a range of different candidate materials that meet a given target. This increases the probability that one or more of the candidate materials will meet the required specifications in a single production test run. Our client estimated that they could save around $90,000 per formulation if they could reduce their production trials from 2-3 runs to just one. Overall, the new active learning tool not only saves money, but provides additional time to further optimize the recipe at the lab scale if needed to meet customer requirements or alternatively bring the new material to market several months sooner. This helps ensure that scale-up can be successful given tight customer deadlines and enables sales growth.