Acceleration of Analysis Pipeline Helped Secure $500M Investment
This cancer therapeutics biotechnology company was on a tight cash runway to deliver on milestones. Enthought’s timely AI/machine learning-driven solution accelerated and operationalized their analysis pipeline, which helped them secure an investment over $500M.
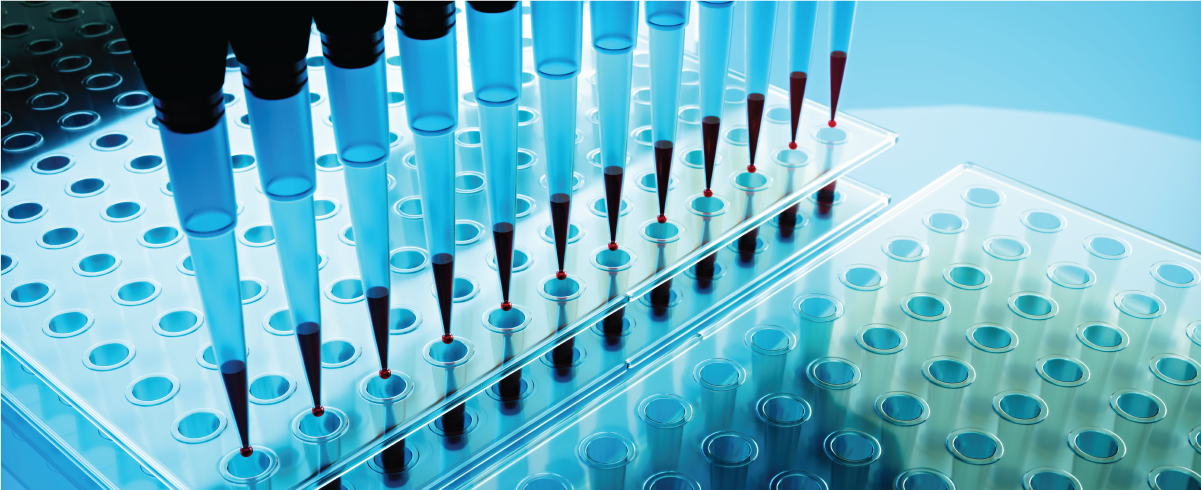
Transforming High Throughput Screening Data into Actionable Insights with Data Modeling and Visualization
A mid-size small molecule cancer therapeutics biotechnology company using a custom high throughput screening (HTS) platform sought to target previously undruggable oncology targets. Their core group of researchers are incredibly motivated and enthusiastically pursuing novel cancer treatments. They came to Enthought looking for ways to accelerate and disseminate their high throughput screening results to enable their researchers to more quickly pursue potential targets. Accelerating their analysis pipeline was also associated with securing the next round of investment, a common milestone in the competitive pharmaceutical space.
Common Challenges Lengthening Time-to-Discovery
The customer's proprietary HTS platform hardware and data capture had recently been operationalized, but analysis of the large amount of data required aggregating information across thousands of files. Simply knowing where a file was in a folder would not provide nearly enough data to analyze the results of a given experiment. The customer developed an analysis routine to extract information from these files, but evaluating the accuracy of this analysis and distributing the results of the complex analysis was still a huge bottleneck. The expert researcher who developed this analysis routine was now also responsible for distributing the interpretation to all researchers rather than enabling researchers to evaluate the experimental results themselves. Further, the team was not able to ask new questions of the analysis results because the experimental data and analysis were not modeled in a way to consistently convey the meaning and context of the data across the organization.
The Limits of Data Management Platforms
The customer came to Enthought seeking a data management solution, but Enthought found that while data management was necessary, it would not be sufficient to achieve the customer's ultimate research and business goals. They ultimately needed a way to rapidly aggregate and visualize all of the experimental data and analyses in ways that any researcher in their organization can use, not just the senior researcher. Enthought's deep understanding of the science and innovative ideation capabilities allowed the customer to think beyond data management and collaboratively develop a plan to transform the way their researchers interacted with the experimental data.
A More Creative Approach for the Iterative Nature of Drug Discovery Research
While data scientists and many platform providers think about data as files and folders, Enthought thinks in terms of data models. What is in those files that describe a scientific experiment or result? What variations of the workflow are being developed, and what new scientific questions are being pursued? How will the results inform the design of the next experiment?
We started by working with the scientists to develop a data model for their experiments and results. We rapidly iterated and collaborated on what the scientists needed to visualize to truly understand the results of the experiment. Our approach centered around experiments and results, not the files and folders. We also knew that simply being able to visualize data to answer today's questions was not enough to be competitive. Labs need to be able to answer tomorrow's questions as well. That is where innovation truly happens. Enthought Edge, a cloud-native scientific workbench purpose-built for R&D, provided the perfect environment for this. We optimized and accelerated analysis of the current workflow by building a tailor-made data dashboard to answer the immediate questions of the scientists. We enabled innovation by building a scientist data API to allow researchers to load their data in analysis-ready data models to programmatically explore and ask new questions using the power of AI and machine learning.
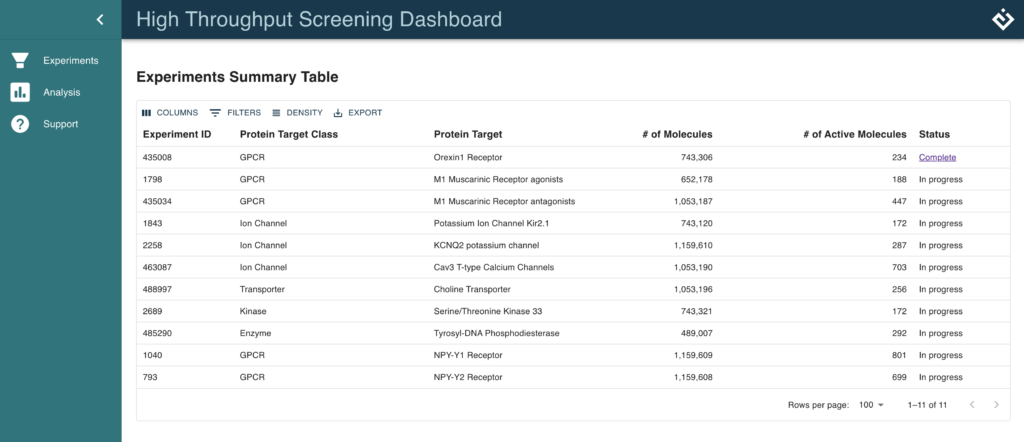
Purpose-Built Solution to Expedite Analyses and Simplify Collaboration
Developing insights from scientific research requires more than simply loading data from a file. It requires connecting the data from many different sources across the experimental landscape. Enthought Edge enabled researchers to filter and visualize the results of their HTS experimental data, while also visualizing the corresponding:
- Original raw data
- Chemical structures and properties
- Quality control metrics
- Population chemical descriptor distributions across the entire compound library
Researchers could then easily share the analyses with colleagues, save a filtered subset of the results for future analysis, and export the results to be further analyzed in the researcher's tool of choice (e.g., Excel, Tableau, Python, R, etc.).
The process of loading these large datasets for exploration is also very memory intensive. Enthought Edge allows users to scale the data dashboard and Jupyter analysis environment to fit the researchers' needs—whether the researcher wants to:
- Load data from multiple experiments to be aggregated and analyzed requiring additional memory
- Work with CPU-intensive clustering algorithms
- Experiment with training machine learning models requiring GPU acceleration
Additional resources can be provisioned by the researcher, and the data previously visualized in the data dashboard can easily be accessed with the scientist-friendly data API.
The customer was further challenged by being in the middle of an IT-led data management transition. There were long-term plans to implement a new, large data lake. However, the needs of the researchers were immediate. The new data lake was important for long-term goals, but such changes are slow to realize. We built import tools and connections to data sources to use the data where it already was. Leveraging Enthought Edge’s Native Application Framework, this thin interface to the underlying data source can easily be changed to use the new data lake when it comes online, and the scientists won’t miss a beat in their research.
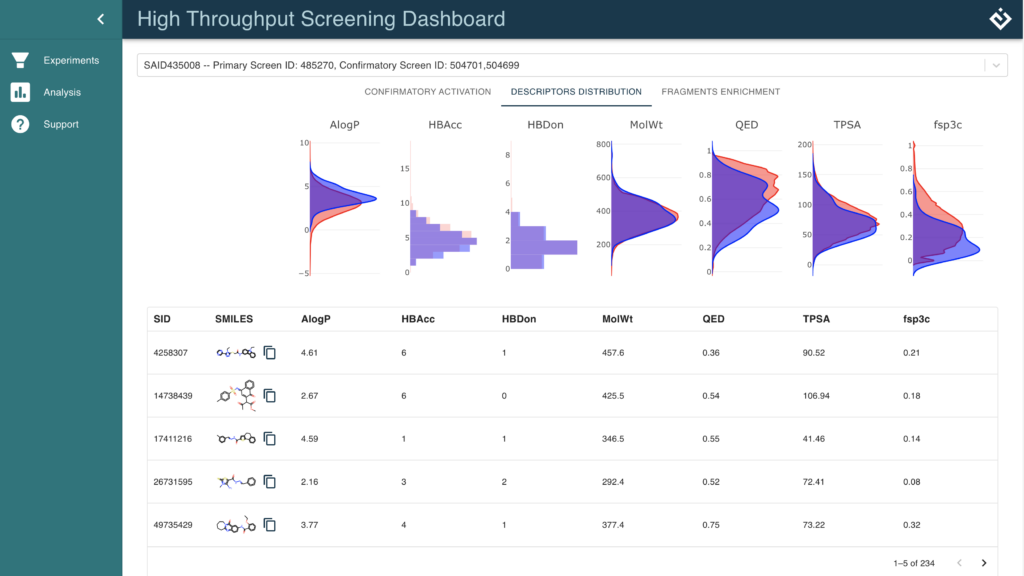
Compare the distribution of molecular descriptors of your hits to the entire compound library.
Acceleration of Analysis Pipeline Helped Secure $500M Investment
As with many biotech companies, this customer was on a tight cash runway to deliver on milestones. Our solution helped them secure a potential investment of over $500M, by accelerating and operationalizing their analysis pipeline, allowing senior researchers to innovate rather than simply maintain and distribute results to the rest of the R&D team.
Share this Case Study
Let's Talk About Your R&D Lab Needs
Contact our Life Sciences Solutions Group to discuss how Enthought can help leverage advanced technologies to solve the challenges in your R&D lab.